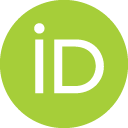
Accepted 11 October 2020
Available Online 24 October 2020
- DOI
- https://doi.org/10.2991/nlpr.d.201013.001
- Keywords
- Sentiment analysis
Natural language processing
Twitter
Tweets
Cosine similarity
Polarization axis
Classification bias
Axis score - Abstract
A new method of approaching sentiment classification is proposed where the likelihood of word embeddings to produce useful information from limited Twitter data is studied. The novelty of this work is in determining how short corpuses (taken from Twitter data) are polarized to multiple axes with respect to a subject, as opposed to using a single positive-negative sentiment axis to classify the text with respect to a subject. The unique methodology of this model focuses on deconstructing a short corpus (microblogging entry from Twitter) into key tokens, identifying the correct axis of the sentiment (the polarization axis) using cosine similarity, and then using this axis to generate polarization values to classify each selection of text into fine-tuned axis values. Results of this study show that a single axis may not be enough to express a sentiment. Various axes will have to be combined for better results. Results were measured in terms of classification accuracy, classification bias, and an axis score.
- Copyright
- © 2020 The Authors. Published by Atlantis Press B.V.
- Open Access
- This is an open access article under the CC BY-NC license (http://creativecommons.org/licenses/by-nc/4.0/).
Cite This Article
TY - JOUR AU - Sikha Bagui AU - Carson Wilber AU - Kaixin Ren PY - 2020 DA - 2020/10/24 TI - Analysis of Political Sentiment From Twitter Data JO - Natural Language Processing Research SP - 23 EP - 33 VL - 1 IS - 1-2 SN - 2666-0512 UR - https://doi.org/10.2991/nlpr.d.201013.001 DO - https://doi.org/10.2991/nlpr.d.201013.001 ID - Bagui2020 ER -